Supply Chain AI Lab
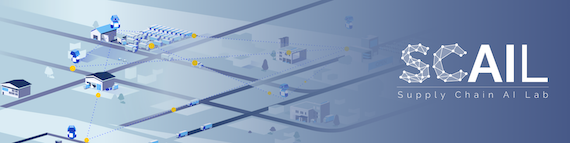
Manufacturing is not all about the predictable and the controllable – factory systems, supply chains, product lifecycle networks are partly emergent, partly designed
complex adaptive systems. The explosion of data, digitalisation and artificial intelligence in manufacturing and supply chains helps us both understand these complex systems and gives us tools to be prepared for the unexpected.
The Supply Chain AI Lab (SCAI-Lab), studies complexity science, emerging artificial intelligence technology, and nature-inspired and agent-based computing techniques to develop novel tools and methods for understanding and handling emergent outcomes in industrial systems.
We create methods to discover hidden patterns in data that yield useful insights for improving supply chain operations. These insights can be used to forecast deliveries, disruptions, find out hidden information, estimate risks, predict quality of goods and even estimate the best price for procurement negotiations. Once the system state has been predicted, then autonomous algorithms can control daily low-level operations to nudge supply chain systems to a more desired state. Some of our current activities include:
- Autonomous supply chains with agent-based systems
- Predicting the formation of “hidden dependencies” in supply networks
- Predicting risk and disruptions in supply networks
- Large-scale real time optimisation of supply network performance, reliability, and resilience
- Digital twins and their interaction
- Study and detection of self-organisation and emergence in supply networks
We also create AI systems that process and analyse data generated within a supply chain system in order to optimise product quality, throughput and utilisation. For example, data from metrology equipment could be used to diagnose likely trends in quality and determine where appropriate interventions should be placed.
New cyber-physical systems in manufacturing result in autonomous systems where decisions are made by a network of interacting agents. Examples include distributed planning of production, and supply chain deliveries and services. We develop analytics solutions to enable such systems to make optimal, on-time decisions using data. These may include collaborative systems that learn from common datasets, and undertake coordinated procurement actions.