Martin Ho is a postdoctoral researcher at the Centre for Science, Technology & Innovation Policy (CSTI) at IfM. He applies network science and systems engineering approaches to quantify evidence on R&D translation and challenge-led innovation, with a particular focus on the biomedical industry and DARPA model of innovation. Martin’s role at CSTI involves working with policymakers and scholars on the question of how best to organize innovation programs to achieve long-term commercial/societal outcomes, and contributed to projects such as Cambridge Industrial Innovation Policy’s roundtable on designing challenge-led innovation programs in UK, Babbage Forum’s industrial innovation policy workshops, the Government Office for Science’s technology capability prioritization project, and Department for Business, Energy and Industrial Strategy’s international R&D spending prioritization. He is also an academic fellow at the Centre for Science and Policy (CSaP) and was Treasurer of the Cambridge University Science and Policy Exchange (CUSPE). Martin’s background in genetic engineering and immunology provides him with an alternative perspective on the interconnectedness of innovation systems. He is driven by the quest to quantify the often intangible pace of innovation, a pursuit balanced by his appreciation for natural complexities.
——————————
Research interests
While developed economies allocate a significant portion (c. 2.5%) of their GDP to R&D, there remains a gap in robustly understanding of how technology emerges and evolves. The journey from an emerging idea to its full technological potential is not well-charted. Current methods of tracking technological progress, whether through regression of performance metrics over time or qualitative assessments at the firm or industry level, fall short. My research addresses this gap by tracing the scientific origins and growth of technologies such as mRNA vaccines, large language models, and quantum computers. I work closely with physicists to develop mathematical frameworks that navigate through a network of publications, patents, clinical trials, regulatory milestones, and other markers of technology maturity. This collaboration allows us to uncover patterns of innovation and identify potential bottlenecks quantitively. This approach not only sheds light on the process of technological evolution but also aims to inform innovation policy and investments with a more strategic vision. Those interested in our findings would include technology managers who wish to benefit from real-time, quantitative intelligence on emerging technologies; and technology investors who carve data-driven evidence to inform budget allocation for a range of uncertain radical technologies.
——————————
Research projects
- Long-term innovation dynamics of EmTech emergence
New technologies emerge when science and existing technologies are applied to fulfill industrial objectives. Given the nonlinearity across research, development, production, and deployment, one knowledge gap is the manner in which different streams of knowledge evolve, combine, or diverge into different technological endpoints. Another lapse is datasets and models that describe the diffusion and translation of innovation. Elucidation of these patterns could diagnose the maturity of emerging technologies and signpost RD&D support according to technological maturities and complementarities. In the meantime, RD&D funding entities, either explicitly or implicitly, make decisions about which technological maturity to operate at. Measuring the technological maturities that funders intrinsically operate at quantifies the intrinsic “division of labor” across entities. This understanding is particularly important in structure mission-oriented innovations which involve maturing nascent concepts/technologies towards clear technical goals across multiple agencies.
- Data- and network science driven revisitation of innovation models
Models that dominate the expectation and analysis of innovation policy, particularly the linear and chain-linked models, have not been quantified or updated since the 1940s. This is because knowledge and the flow of it are understood as intangible. The availability of relational databases, citation data, innovation markers, computational resources to analyze them allows the quantification of variables within incumbent models of innovation and place a value and frequency on knowledge transmission. Revisiting and validating models of innovation through statistics would assess the utility of these thinkings for contemporary innovation. This will test whether innovation policies are aligned with the right expectations. While the complexity of technological emergence forbids its prediction, the study of innovation networks through the use of graph theory mathematics open opportunities to defining technological certainty. The correlation of many these network variables over many technological datasets might allow reliable complex system models that can provide probabilistic forecasting of technological emergence. These models can be combined with other strategic foresight technique to inform prospective RD&D resource allocation. The ability to find reliable signals, if not forecast, technological emergence would increase preparedness in innovation policy. Beyond policy, effective technological emergence signals would also revolutionize RD&D investment decisions and in venture capitals.
- Evolving social network of innovation communities
Agglomeration of scientific participants promotes scientific discovery and dissemination. Building on network analysis of social networks, e.g. in social media, the composition, centrality, and connectedness of innovators and funders can be dynamically defined over technological maturities. Understanding the evolution of entities coalescing an innovation trajectory inform the structuring of innovation policies and infrastructure that promote these communities. For instance, it is widely believed that large-scale, “diffusion-oriented” agencies such as the UKRI and NIH favor basic research while firms and other smaller specialist agencies support innovation in the downstream; whereas the funding pattern of “mission-oriented” innovation agencies such as DARPA and ARIA is rarely measured. There is little analysis of the way in which support of innovation is distributed over time, and over entities — a gap this project addresses.
- Large language models for international comparative analysis of innovation policies
Large language models (LLMs) may drastically reduce workloads of innovation policy analysis. However, very few LLMs are fit for purpose for analyzing policy documents and other gray literatures. Using open source tools, this project augments existing LLM workflows and modules to realize workflow reduction for policymakers and analysts while preserving accuracy and avoiding pitfalls of first-generation generative AI models, such as hallucinations. These advanced models are adept at processing and synthesizing complex datasets and gray literatures to identify trends, patterns, and gaps within the innovation policy landscape. By automating the analysis of large volumes of data, LLMs enable stakeholders to rapidly digest and act on insights, which is critical in the fast-paced nature of emerging technologies.
- Strategic technology roadmapping for challenge-led innovation / DARPA-clones
How can governments assess the contribution of evolving technologies to evolving socioeconomic goals? While such situations in the private sector typically command backcasting approaches, in national technology strategy contexts, conventional methods are uniquely challenged by institutional complexity, extremely long time horizon, technological uncertainty, and technological interdependence. This project qualitatively adapts traditional roadmaps and quantifies the architecture of technology roadmaps through network science and AI/ML. Technology roadmaps visually shows technologies that is used by current or future products and is materially a network of dependencies among technologies and products with timestamps. To analyze complex systems, intermediary layers with hierarchical decompositions are added between technology and product layers in a
roadmap. Conventional roadmapping architecture can be reconfigured to explore, ab initio, multiple potential technology-agnostic pathways that would achieve the mission in the form of shortest calendar time and longest network path. We analyze two innovation programs in the roadmapping view: A
same roadmap is presented in Gantt chart, dependency structure matrix (DSM), and directed acyclic graph (DAG) to interrogate dependencies among basic, applied, proprietary knowledge, manufacturing, and commercialization at increasing granularity. We then compare and contrast task parallelization informed by critical path, clustering, and longest path methods.
——————————
Recent publications
An updated list is available at https://scholar.google.com/citations?user=WnGED28AAAAJ&hl=en
Martin Ho, Henry CW Price., Tim S Evans and Eoin O'Sullivan. Enhancing foresight models with network science: Measuring innovation feedbacks within the Chain-Linked Model. Technological Forecasting and Social Change, 213, (2025). https://www.sciencedirect.com/science/article/pii/S0040162525000411
Martin Ho, Henry CW Price., Tim S Evans and Eoin O'Sullivan. Who made the mRNA vaccine? Measuring division of labor in therapeutic innovation. Nature Biotechnology, 42(11), 1643-1648, (2024). https://www.nature.com/articles/s41587-024-02441-7
Martin Ho, Henry CW Price., Tim S Evans and Eoin O'Sullivan. Dynamics of technology emergence in innovation networks. Scientific Reports 14, 1411 (2024). https://www.nature.com/articles/s41598-023-50280-4
Martin Ho, Henry CW Price., Tim S Evans and Eoin O'Sullivan. "Morphology of Vaccine RD&D translation." arXiv preprint arXiv:2310.18193 (2023). https://arxiv.org/abs/2310.18193
Martin Ho, Henry CW Price., Tim S Evans and Eoin O'Sullivan. "Order in Innovation." arXiv preprint arXiv:2302.13076 (2023). https://arxiv.org/abs/2302.13076
Martin Ho, Elizabeth Killen and Eoin O’Sullivan. The challenges of challenge-led research and innovation agencies (2021). https://www.ciip.group.cam.ac.uk/reports-and-articles/the-challenges-of-challenge-led-research-and-innovation-agencies/
——————————
Teaching
I currently supervise two Industrial Systems, Manufacture and Management (ISMM) Master’s students on using technology foresight methods to operationalize challenge-led innovations.
——————————
Education
- PhD in Engineering, University of Cambridge — Supervised by Dr. Eoin O’Sullivan and Dr. Rob Phaal; Examined by Prof. Giovanni Dosi and Prof. Alexandra Brintrup — achieved in two years
- MPhil in Technology Policy, University of Cambridge — Top of class
- BSc in Biochemistry and Biotechnology, Imperial College London — First class
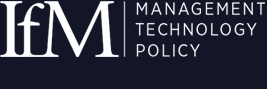
- Institute for Manufacturing
- 17 Charles Babbage Road
- Cambridge CB3 0FS
Research
- Artificial Intelligence
- Asset Management
- Business Model Innovation
- Computer Aided Manufacturing
- Decision-Making for Emerging Technologies
- Design Management
- Digital Manufacturing
- Distributed Information & Automation Laboratory
- Ecosystems, Platforms & Strategy
- Fluids in Advanced Manufacturing
- Healthcare
- Industrial Photonics
- Industrial Resilience
- Industrial Sustainability
- Inkjet Research
- Innovation and Intellectual Property
- International Manufacturing
- Manufacturing Industry Education Research
- NanoManufacturing
- Science, Technology & Innovation Policy
- Strategy and Performance
- Technology Enterprise
- Technology Management
- Service Alliance
- University Commercialisation and Innovation Policy Evidence Unit