The power of AI for supply chain efficiency
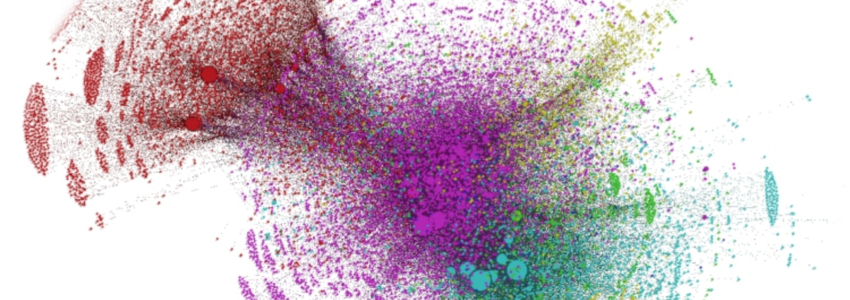
Artificial Intelligence (AI) offers huge potential for supply chains, helping to reduce disruption and improve efficiency. So far, however, there has been a lack of practical examples and case studies demonstrating how data-driven approaches can be implemented effectively. Research from the IfM’s Manufacturing Analytics research group, led by Dr Alexandra Brintrup, has been addressing this gap by running pilot studies and highlighting the opportunities and potential pitfalls. Alexandra explains what her research reveals and the lessons for organisations…
Supply chain analytics isn’t a new concept – in fact the manufacturing sector has long been an enthusiastic adopter of data-driven techniques. So apart from a fancy new name, what is really new?
A combination of three emerging developments is changing the game: The first is computational power, enabling us to do calculations real time; secondly, powerful new algorithms can automate analysis and decision-making; and crucially, new and previously untapped sources of data are emerging.
‘Supply chain analytics’ is an umbrella term, referring to a multitude of capabilities. There is no single solution that works for every organisation – it depends on the nature of the supply chain, the organisational strategy and priorities, and the information that is available. And not every capability is appropriate for every organisation – they should not be viewed as a to-do list! Instead, firms can pick and choose which capabilities to develop to suit particular supply chain functions and business needs.
However, there are some common questions that organisations can address in order to understand how supply chain analytics and AI can best be deployed in their own context.
How much data and from what source?
Where does your data come from? Traditionally in supply chains we’ve had enterprise resource planning (ERP) systems taking structured sources of data (which are mostly manually populated but also drawn from some automated processes). Now we have data from a larger array of sources: smart products that provide data with status updates on their use, location, and condition, through sensors and IoT connectivity; GPS location tracking data; and even unconventional sources such as social media.
Much of this data can be obtained in real time, and can be gathered from beyond your immediate organisational boundaries – whether from supply chain partners, external organisations or customers.
How will the data provide valuable information?
Data volume can be overwhelming, so understanding and focusing on what will genuinely add value is essential. How will you use the data to create a better understanding of what is going on?
It’s also important to consider how data from different sources can be integrated to provide a dynamic overview. This can, for example, enable predictive analytics to reduce disruption.
How does the data improve decision-making?
Improved data availability offers the potential for much greater awareness of systems. But what will you do with the new-found awareness - what kinds of decisions will the data be used to improve?
Can you identify ways to optimise current processes, or about how to redesign systems in the future? Is the focus primarily on streamlining day-today operations, or on tactical areas, or strategic decisions? And can data be used collectively across the supply chain to improve efficiency?
How does this support automation or semi-automation of tasks?
Data analytics can allow hidden patterns and trends in the data to be uncovered and acted upon, in order to improve supply chain operations. The automation or semi-automation of mundane operational tasks, identified through the data, can have a transformative impact on optimisation in the supply chain.
Supply chain analytics in practice: Real-world examples
Our Manufacturing Analytics research team has conducted several studies on supply chain analytics with partners from the automotive, and aerospace industries as well as FMCG and other sectors.
The goal was to map the supply chain structure, understand how disruptions may cascade and impact this structure, and then use this knowledge to inject resilience after predicting hidden dependencies and supplier deliveries (see Figure 1).
Figure 1: Identifying and reducing disruption in the supply chain through data analytics
These studies reveal five key lessons for using supply chain data analytics:
1. Don’t underestimate the power of descriptive analytics to lead to more realistic solutions
The very first lesson is about not underestimating the potential benefits that can be gained from exploratory, descriptive analytics before moving onto solution finding. The insights we gained from exploratory studies provided greater depth than we expected. For example, the hub-spoke structure that emerges in supplier-manufacturer connections and the density of connections told us that there is a higher than expected likelihood of tier one suppliers connecting to one another, unbeknownst to the original equipment manufacturer (OEM).
By mapping the supply chain data, we were able to spot patterns, and predict what failures were likely to occur, and therefore take much better precautions. In one of the studies we worked with a large FMCG corporation, and we able to spot where inventory could be injected to provide a buffer against likely stock shortages. In fact, we found that some types of network structures require much less inventory to ride out the same level of disruption - so we could map out what level of inventory is needed for a particular type of network structure to reduce disruption without unnecessary stockpiling.
2. Don’t go crazy! A minimalist approach may yield the best results
The second lesson is about staying focused and avoiding overcomplicated solutions. For example, one company we worked with wanted to find out which of their suppliers were supplying to each other – as this is a problem for reliability of supply. Where were there hidden dependencies amongst suppliers? Initially we tried to identify this with sophisticated methods: using time series data, and trying to tease it all out with deep neural nets, recursive nets, and other techniques… but nothing worked.
Then we went to back to basics, and asked ‘What is likely to connect suppliers to each other?’ If they produce these products, maybe their models are compatible, so they supply the same OEMs. This worked. Getting back to fundamental patterns was key.
3. One solution doesn’t fit all
Often data across supply chains can quickly become too complicated. In our studies, variables such as suppliers, parts, delivery times, volumes, locations, routes, level of confidence – all added up to a very large data set that became too complex. We found that it was essential to divide the problem into more digestible chunks, considering one or two elements at a time to produce more meaningful and useable information.
4. Domain knowledge is golden
It is crucial to work with the operational team to decipher patterns in data – for example in a supplier disruption prediction project, what we initially thought was noise in the data turned out to be new product configurations, which helped us understand how the system may stabilize over time.
5. Strive to create traceability, accountability and buy-in
The fifth and final lesson? It’s about trust. AI is wonderful, a life-long passion of mine, but it’s clear that not everyone trusts AI. And they are right to ask these important questions. When it goes wrong, who will be accountable? Can we place mechanisms to make it transparent? These are big questions without straightforward answers, at least for now.
In our research, we worked with an aerospace company to create a self-organizing system using what we call ‘software agents’ (essentially what drives Alexa and Siri) to automate spare parts procurement. The system would take data from sensors, analyse them to understand what part is expiring or deteriorating by when, then find the best supplier to schedule aircraft maintenance depending on when and where it is flying. This is a complicated problem, and the analytics could provide optimal solutions. It could even negotiate with suppliers and run auctions.
But the questions asked by the real people involved were pertinent: Exactly how do you arrive at these solutions? What if our people want to negotiate with suppliers themselves? Are you automating me? So due to the valid questions raised about trust, the solution that had been developed was patented but will take a lot longer to be implemented.
The key lesson perhaps is that we need more research to build transparency of algorithms and understand how and when they should be used.
We are currently working with several companies to understand how these concepts can be applied to a variety of sectors. Please contact Alexandra Brintrup: [ab702@cam.ac.uk] to find out more.
For further information please contact:
Dr Alexandra Brintrup